A breakthrough in image recognition of clover allows for optimised nitrogen supply to grass clover pastures
Using machine learning, researchers from Aarhus University have developed a method that can map the distribution of grass, clover, and weeds on the farmer's fields, so that he more easily can adapt the supply of nitrogen. The method uses so-called DeepLabv3+ neural networks, and it is extremely robust and allows coverage of large areas in a short time.
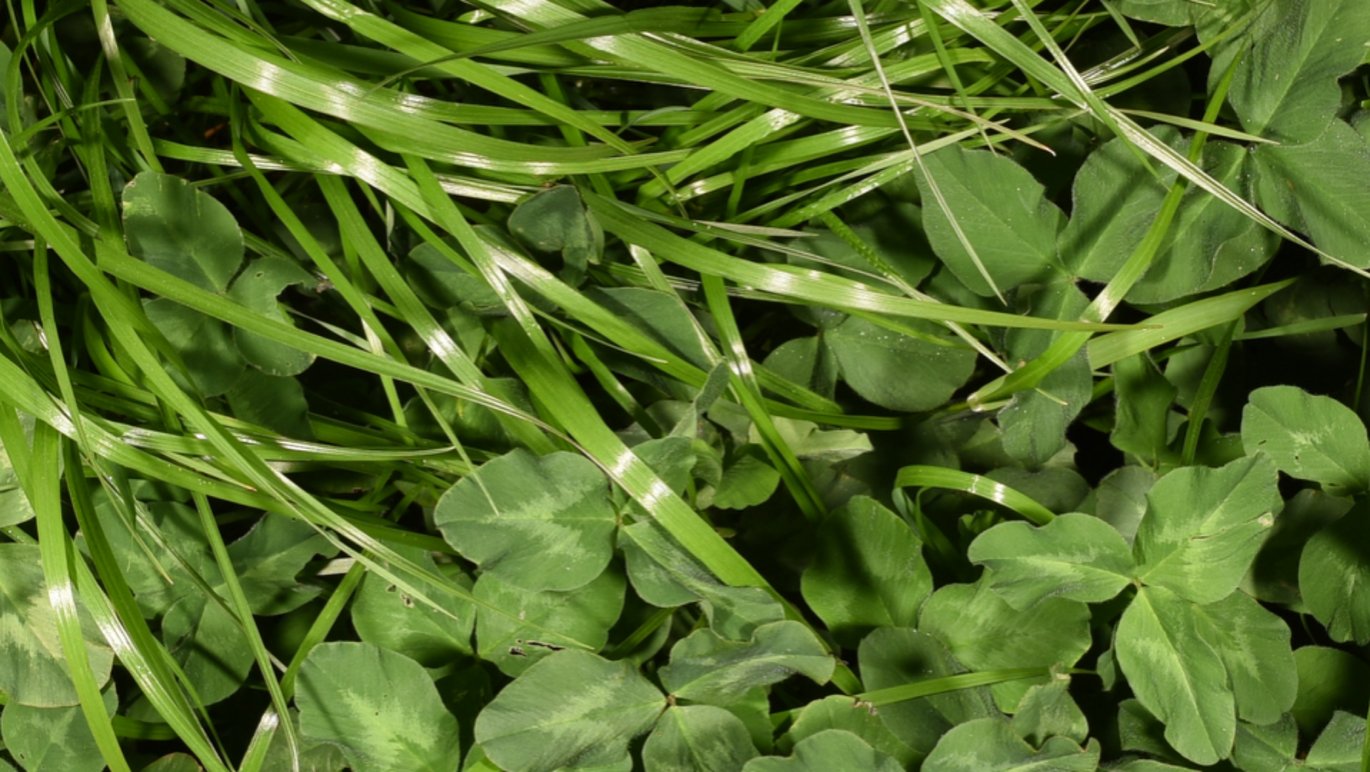
It is complicated to optimise nitrogen supply for clover grass. Grass on one hand has a very high nitrogen requirement, while clover on the other has no need. Therefore, the optimal supply of nitrogen depends to a large extent on the clover share in the field. Differences in growth conditions give variation in clover shares from place to place, year to year, and during one year. In addition, there can be large local variations within the field, which means that the need for fertiliser can be very different even within small areas. All this combined has made it almost impossible for the farmer to calculate the right amount of fertilizer.
For many years, there has been a focus on developing camera solutions that can help in this situation. But despite many efforts, it been difficult to translate the research into practice - at least until now," says professor and section leader Jørgen Eriksen from the Department of Agroecology at Aarhus University.
Can tell the difference between grass and clover
The researchers have used a so-called DeepLabv3+ model, which is trained to know the difference between grass, different clover species, weeds, and soil in images under different weather conditions (sun, rain, and dew). To test the model, it has been used to determine the clover share of a total of 915 plots spread over three seasons and at four sites with varying conditions in soil type, climate, and yield level.
“Our model is able to predict the actual proportion of clover, which in the experiments was determined by a simple botanical analysis. That is, the plant species had been sorted and weighed. And the model could predict the proportion with an explanatory degree of an impressive 91%, and for mixtures only with grass and white clover the explanatory degree was 95%,” says scientific assistant Søren Kelstrup Skovsen from the Department of Electrical and Computer Technology at Aarhus University.
This is a significantly higher degree of explanation compared to previous methods, where generalisation across locations and seasons in particular has been problematic.
"With the introduction of deep learning and data-driven image recognition in clover grass, we have seen a huge leap in generalisation, and with the latest DeepLabv3+ model, accuracy has been given yet another boost," says scientific assistant Søren Kelstrup Skovsen.
High image quality is important
The success of the DeepLabv3+ model can not only be attributed to advances in model development, but is to a large extent also related to high and consistent image quality.
"By controlling the framework for image collection, we have ensured that the model always has the homefield advantage. This not only provides higher accuracy, but also increases the reliability of the system in the real world," says Søren Kelstrup Skovsen.
Effective image collection is very important if the DeepLabv3+ model is to be used for mapping fields based on up to 200 images per hectare.
”That is why we have used an ATV-mounted camera, which is specially developed for collecting high-quality images even at speeds of up to 50 km / h. In our experiment, we have thus managed to collect 29,848 images, spread over 16 clover grasslands, with approx. 17 acres per hour. And due to the automatic image recognition of each image, we were able to demonstrate the method by accurately mapping the clover share of a total of 225 hectares," says Søren Kelstrup Skovsen.
Possible to optimise the fertiliser application
DeepLabv3+ can thus be used to map the distribution of clover, grass, and weeds in fields at a speed of up to 17 hectares per hour.
“It is really a breakthrough in relation to the farmer's ability to optimise the fertiliser supply in the individual clover grassland based on the proportion of grass and clover. Our goal is an optimised nitrogen supply for the benefit of both feed quality, economy, and the environment, and the development of this extremely robust model helps to make this possible by simply using a camera mounted on an ATV, tractor, mower, or even a drone,” says Jørgen Eriksen.
Behind the research |
---|
Collaborators: Department of Electrical and Computer Technology at Aarhus University and Department of Agroecology at Aarhus University |
Funding: Innovationsfonden (Smartgrass, 6159-00001B) and GUDP (Cloversense, 34009-16-1088) |
Read more: You can read the article ”Robust Species Distribution Mapping of Crop Mixtures Using Color Images and Convolutional Neural Networks”. It is published in Sensors, and it is written by Søren Kelstrup Skovsen, Morten Stigaard Laursen, Rebekka Kjeldgaard Kristensen, Jim Rasmussen, Mads Dyrmann, Jørgen Eriksen, René Gislum, Rasmus Nyholm Jørgensen, and Henrik Karstoft. The publication is part of a larger project called SmartGrass, which has a large group of partners, the publication here is authored by researchers from AU and is the researchers' own product. |
Contact: Professor Jørgen Eriksen, Department of Agroecology, Aarhus University. Tel.: 51680554. Mail: jorgen.eriksen@agro.au.dk. |